Metallurgical Abstracts on Light Metals and Alloys vol.54
Globularization and Recrystallization of a Ti-6Al-2Sn-4Zr-6Mo Alloy under Forging in the (α+β) Region: Experiment, Phenomenological Modeling and Machine Learning
Hiroaki Matsumoto*
*Faculty of Engineering and design, Kagawa University
[Published in ISIJ international, Vol. 61 (2021), pp. 1011–1021]
https://doi.org/10.2355/isijinternational.ISIJINT-2020-598
E-mail: matsumoto.hiroaki[at]Kagawa-u.ac.jp
Key Words:Ti-6Al-2Sn-4Zr-6Mo alloy; dynamic globularization; dynamic recrystallization; Avrami model;
machine learning; finite element simulation.
The Ti-6Al-2Sn-4Zr-6Mo (Ti-6246) alloy is an α+β titanium alloy with good combination of strength, toughness, high temperature creep property and crack growth resistance. This work examined the dynamic microstructural conversion behavior (specifically focusing on dynamic globularization of α phase) occurred under hot forging process (at temperatures ranging from 750°C to 1050°C) of the Ti-6246 alloy with various kinds of starting microstructure. Plastic flow behavior of the microstructure having the α-lamellae exhibited a peak stress followed by continuous flow softening due to frequent occurrence of lamellae kinking and globularization of the α phase. For the microstructural conversion of β phase, continuous dynamic recrystallization (CDRX) was dominantly occurred. And the enhanced CDRX is observed for the lamellar starting microstructure as compared to the bimodal and the equiaxed starting microstructures. On the globularization kinetics according to a phenomenological Avrami approach and a machine learning approach, when the processing conditions were categorized into 3 groups from a hierarchical clustering, the estimated globularization fraction from Avrami approach corresponded reasonably well with the experimental data only in a specific process condition group in which corresponded with a higher temperature and a higher dynamic globularization fraction. Thus, this work points out the possibility of a reliable prediction of dynamic globularization according to an optimal combination of the Avrami approach and the machine learning approach.
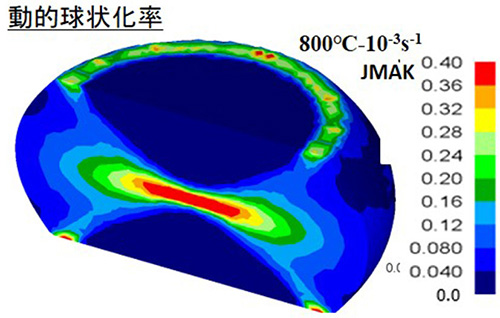
Distribution of dynamic recrystallization fraction by FEM, which is established by dynamic globularization model in terms of JMAK model.