Metallurgical Abstracts on Light Metals and Alloys vol.55
Strength and Ductility Balance of a Ti-5Al-2Sn-2Zr-4Cr-4Mo (Ti-17) Alloy with Various Microstructures: Experiment and Machine Learning
Hiroaki Matsumoto*, Daiki Tadokoro** and Irvin Séchepée***
*Faculty of Engineering and design, Kagawa University
**Graduate Student, Kagawa University, 2217-20 Hayashi-cho, Takamatsu, Kagawa 761-0396, Japan.
(Now belonging to Hitachi Metals, Ltd., 2107-2 Yasugi-cho, Yasugi, Shimane 692-8601, Japan)
***Graduate Student, Kagawa University, 2217-20 Hayashi-cho, Takamatsu, Kagawa 761-0396, Japan.
[Published in ISIJ international, Vol. 61 (2021), pp. 2844–2854]
https://doi.org/10.2355/isijinternational.ISIJINT-2021-261
E-mail: matsumoto.hiroaki[at]Kagawa-u.ac.jp
Key Words: Ti-5Al-2Sn-2Zr-4Cr-4Mo alloy, microstructures, tensile properties, precipitation hardening, machine learning
This work examined the tensile properties and hardness of a Ti-5Al-2Sn-2Zr-4Cr-4Mo (Ti-17) alloy having various morphology of an equiaxed, a (α+β) bimodal, and a (α+β) lamellar (with or without secondary α precipitates) microstructures in terms of an experimental analysis and a machine learning approach. Among the various morphology, a good balance on strength and ductility is obtained in the bimodal morphology. On the strength, higher strength is exhibited in the microstructures having secondary lamellar α precipitates. Here, we experimentally find that the primary α phase of an equiaxed grain or a lamellae acts as only the strengthening according to the rule-of-mixtures, on the other hand, the secondary lamellar α precipitates act as precipitation strengthening dominated by cutting mechanism. On the ductility, it is strongly affected by the difference in morphology and the formation of secondary lamellar α precipitates; high ductility is obtained in an equiaxed grained morphology and in the microstructure with a decreasing fraction of secondary α precipitates. A machine learning approach adapting neural network and clustering (the Ward’s method) algorithms successfully predicts the linking between the microstructural factors and the tensile properties of strength, elongation to fracture and reduction of area; a similar dominant mode to the experimental results is estimated from a machine learning approach. Additionally, a quantitative analysis on the effect of each microstructural factor is successfully estimated according to the sensitivity of variable’s importance in terms of the neural network algorithm.
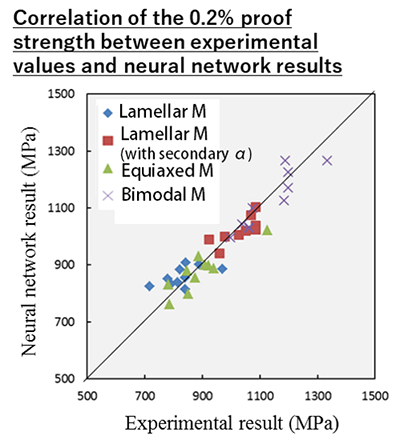
Comparison of the 0.2% proof stress between the machine learning (neural network) result and experimental result (Ti-17 alloy with various kinds of microstructures).